2020 MAY WELL turn out to have been a watershed year in biochemistry and biology. Despite the failure of the establishment in finding a quick cure for the Wuhan-originated coronavirus pandemic, there was the Nobel Prize won by Emmanuelle Charpentier and Jennifer Doudna for CRISPR-Cas9, a gene-editing mechanism with immense possibilities.
Then there is the amazing news in biology of the apparent success of DeepMind’s AlphaFold2 in predicting the shape of proteins with unparalleled accuracy. This has been a quest in biochemistry for at least 50 years, and the fact that a machine-learning algorithm has been able to do it with better than 90 per cent accuracy is truly impressive.
Why is this important? Proteins are the building blocks of life, in a truly literal sense. Enzymes, hormones, antibodies, neurotransmitters, muscles, bones: all of them either consist entirely of proteins or have large quantities of them. Even the spike on a Covid-19 virus, which enables it to attach to a receptor on a cell and invade it, is a protein.
Proteins are long chains or sequences of tens of thousands of amino-acid molecules. And there are 20 types of amino acids.
That means the possible number of proteins is enormous. DNA has only four types of basic nucleotides, and that is enough for massive complexity, as seen in the human genome project. What is truly remarkable is that it is the shape of the protein that matters, not necessarily the specific amino acid in the sequence. More on that later.
First, a thought about Artificial Intelligence (AI) and machine learning (ML). There have been several remarkable outcomes in AI/ML in the recent past: DeepMind (a company owned by Google) itself defeated the world champion at Go, a game with simple rules but significant strategic complexity.
GPT-3 from OpenAI, a California company, is now able to produce astonishingly lifelike texts based on a few samples of someone’s writing; and now AlphaFold2 has performed the feat of predicting the shape of proteins. There is also an ongoing effort at the Massachusetts Institute of Technology to identify those with the coronavirus merely based on the sound of their cough.
There are also glitches on the way, of course. There is a brand-new scandal about Google firing AI researcher Timnit Gebru (a Black woman) allegedly for criticising the huge carbon footprint of crunching large data sets and for highlighting recurring instances of racial and sexual bias in large datasets, which end up causing the algorithms themselves to appear biased.
The thing that we all need to keep in mind is that AI/ML is a performing monkey. It may go through the motions and execute what appear to be wondrous feats, but it simply has no understanding of what is going on: it is an idiot savant, if you want to be more charitable. It is about syntax, not about semantics.
We are not quite there yet, but the excitement over Google’s Alphafold2 is because it might be able to identify precise counter-weapons, based on the shape of the enemy’s own weapons, that can fend off the enemy. Instead of trial and error, if Alphafold2 could narrow the field down to a handful of possible drugs and vaccines, that would be a major boon
Share this on 
AI/ML is merely doing a statistical analysis of correlation, with no idea about causation. Is this enough? Yes, in the real world, to a significant degree of abstraction, this is mostly what we need. To put it bluntly, it is engineering, not science.
Engineers are bothered about what works, not necessarily why it works: that is the province of science. There are not too many theological battles in engineering, unlike in science where deeply held beliefs (often like blind faith) cannot be changed: whence Max Planck’s epigram that “science advances one funeral at a time”.
In a way, all of us are votaries of this engineering philosophy. For instance, unless you are a stylite-type hermit somewhere, you are almost certainly using software of some kind. Even if buggy, all of this software works more or less correctly most of the time, which is all we need. We don’t ask for formal proof of correctness, which would be extremely tedious (unless machines do the proving for us).
Similarly, allopathy (modern, mainstream medicine) is also about that which works. Since it really doesn’t have a theory of disease (except for Louis Pasteur’s germ theory that it adopted as late as the 19th century), it is about using whatever means are at hand to combat whatever illness is about (thus the extraordinary, and in my opinion, dangerous, fuss over the coronavirus vaccines).
Thus, AI/ML works in certain limited domains, and we accept it as useful. Maybe another analogy is helpful: Newton’s physics is not complete, nor does it work in the realms of the very small (subatomic particles) or very large (galaxies). But it is a good enough approximation for everyday use.
Second, the question of aesthetics. There has always been a philosophical question as to whether beauty matters. Even though most engineers and scientists have been trained to think that that is a frivolous question best left to dreamy artists and philosophers, the fact is that elegance and, yes, beauty matters in almost everything.
In the world of software, which is just about the most prosaic thing you can think of, programmers derive quiet satisfaction from writing beautiful code. It is possible to write very ugly code (which even the writer can scarcely bear to look at again), but once you see beautiful and elegant structures, it is hard to go back to the old style. The old monolithic style of writing code contrasts sharply with the spare elegance of Unix, and that trend has percolated to Linux, Android and iOS.
Almost all of us are moved, sometimes to tears, by beautiful things. The fact that this is so suggests that there is evolutionary value to the appreciation of beauty, or else it would have been extinguished as a useless trait somewhere down the line.
Beauty, apparently, is not optional, but integral.
In the Indian tradition of aesthetics and rasas, the importance of structure in invoking certain emotions is well articulated; indeed, one of the cornerstones of Carnatic music is its mathematical precision (this is true of Western classical music as well).
Another example is in the precise utterance of Sanskrit mantras (which, according to the theory, create specific sound patterns that have beneficial effects, and so chanting them in translation in a different language wouldn’t have quite the same effect) that may resonate with certain frequencies in the human body or in the environment. Another example is the great length to which traditionally oral renditions of scriptures went to preserve exactness. Pada paatha, using hand mudras as error-correcting codes for ensuring absolutely correct transmission of the Rig Veda, is a tradition from Kerala.
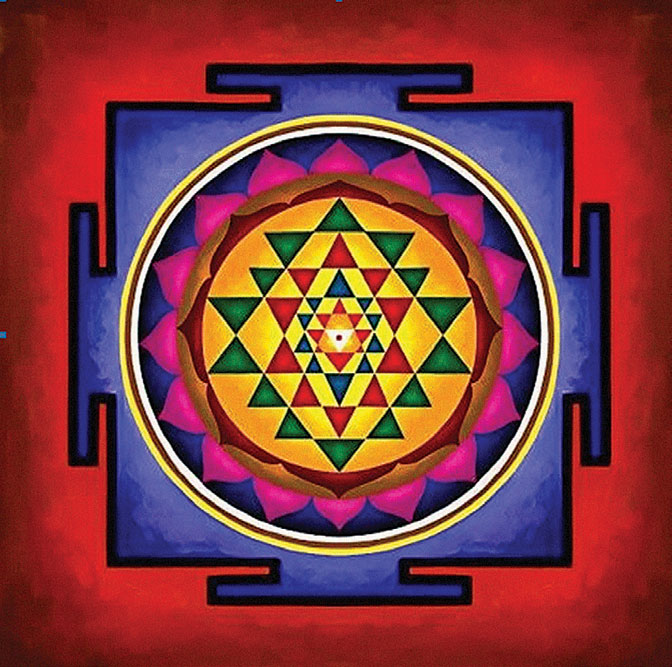
A sri yantra is another example of a structure that is beautiful, precise and quite likely recursive and fractal. Fractals are found so widely in nature that it is likely that our sense of aesthetics can zero in on that property of structures, both human and natural.
Then there is the humble kolam or rangoli. It is astonishing to watch housewives in Tamil Nadu casually and effortlessly create a pattern that is recursive and often fractal, often while they are chatting away with somebody else.
The fractional dimensions of these patterns may have a relationship to theoretical insights, such as Subhash Kak’s conjecture that gravity can be explained if the universe is e-dimensional, where e = 2.71828… , the irrational number called Euler’s constant.
What does all this mean in the context of protein folding? It turns out that a protein, which, as mentioned earlier, is a long chain of amino acids, can be folded into an astronomical number of possible shapes when it is created.
Proteins, according to a podcast from the New Scientist magazine, are the workhorses of biology, and they can do things as varied as being a generator of energy and being a transporter of goods. They are specialised to do these tasks, and that specialisation includes their shapes as well.
Apparently the number of permutations for folding the protein is of the order of 10e300, enormously greater than the number of atoms in the universe, which is supposed to be around 10e80. That would make the task of computing the permutations essentially NP-complete, that is, not computable using brute-force methods. You need certain heuristics or rules of thumb to reduce the universe of possibilities to a manageable number.
What AlphaFold2 has done is to develop its own set of heuristics, a standard ML practice, by reviewing a very large dataset of previously known proteins and their structure. Based on this, it can predict, with a degree of statistical probability, what the structure of a newly formed protein will be. Obviously, that is useful, because the traditional chore of hand-analysing structures through X-ray crystallography is painful, slow and expensive.
A sri yantra is an example of a structure that is beautiful, precise and quite likely recursive and fractal. Fractals are found so widely in nature that it is likely that our sense of aesthetics can zero in on that property of structures, both human and natural
Share this on 
There are very interesting implications. For instance, it is now well-known that the way a virus attacks a normal cell is by matching its ‘key’ to a ‘lock’ in a receptor in the cell. In other words, it has created a structure that matches the ‘keyhole’. In the case of the coronavirus, the spike proteins on its surface are the keys that match the ACE2 receptors in the cell.
Therefore, can we find something that can specifically look for the spike proteins on the surface of the coronavirus, or SARS-CoV-2, using its ‘key’, attach itself to the virus and destroy it? That would be the perfect way of finding a cure, or a vaccine, for the disease.
There exists a vast pharmacopoeia of drugs, tried and tested. And a large number of vaccines too that have stood the test of time. An expert such as Gobardhan Das has suggested testing existing vaccines, such as the BCG vaccine for tuberculosis and a leprosy vaccine, as possible preventives for the coronavirus, based on his hunches about their molecular biology.
We are not quite there yet, but the excitement over Alphafold2 is because it might be able to identify precise counter-weapons, based on the shape of the enemy’s own weapons, that can fend off the enemy. Instead of trial and error, if Alphafold2 could narrow the field down to a handful of possible drugs and vaccines, that would be a major boon.
None of this may happen for years, but it is a promising way forward. There are further complications: it is also necessary to consider how protein molecules interact with each other and with other molecules, say water, in the vicinity. There are 180 million protein sequences known to scientists according to The Economist, but only some 170,000 have had their structures determined so far. Automating the task will help enormously.
The drug discovery time can be reduced; in a future pandemic, researchers may find an antidote among known drugs and vaccines in days, instead of spending months inventing new things and rushing them to market barely tested. That would reduce the risks for humanity and would be a great contribution to public health.
About The Author
Rajeev Srinivasan worked at Bell Labs and in Silicon Valley for many years. He writes widely on the impact of technology on society
More Columns
‘Colonialism Is a Kind of Theft,’ says Abdulrazak Gurnah Nandini Nair
Bill Aitken (1934 – 2025): Man of the Mountains Nandini Nair
The Pink Office Saumyaa Vohra